Multiple myeloma is a relatively rare type of blood cancer that causes uncontrollable multiplication of unhealthy plasma cells in the bone marrow. These cancerous plasma cells crowd out healthy ones and can severely inhibit the body’s immune response to infection.
As part of the Multiple Myeloma Research Foundation, we are contributing to the creation and annotation of one of the largest single cell datasets available for a single hematologic cancer. Our lab alone has helped sequence and align over one hundred individual CD138- bone marrow aliquots from patients diagnosed with Multiple Myeloma, and will be actively processing more over time.
We are working with the Immune Atlas Consortium under the MMRF, with partners at Beth Israel Deaconess Medical Center, Mayo Rochester, Mount Sinai School of Medicine, and Washington University in St. Louis, to characterize the bone marrow immune landscape of Multiple Myeloma from over 300 processed bone marrow aliquots. As an initial analysis, we are trying to establish and understand the baseline differences in immune composition and expression profiles between patients who go on to rapidly progress versus those with sustained non-progression. We are performing similar analyses accounting for multiple other factors, such as cytogenetic risk derived from whole genome sequencing, race, gender. Ultimately, we hope to better understand the factors within the immune environment which explain the rate of progression and success of therapy among newly diagnosed multiple myeloma patients.
Lab Members Involved:
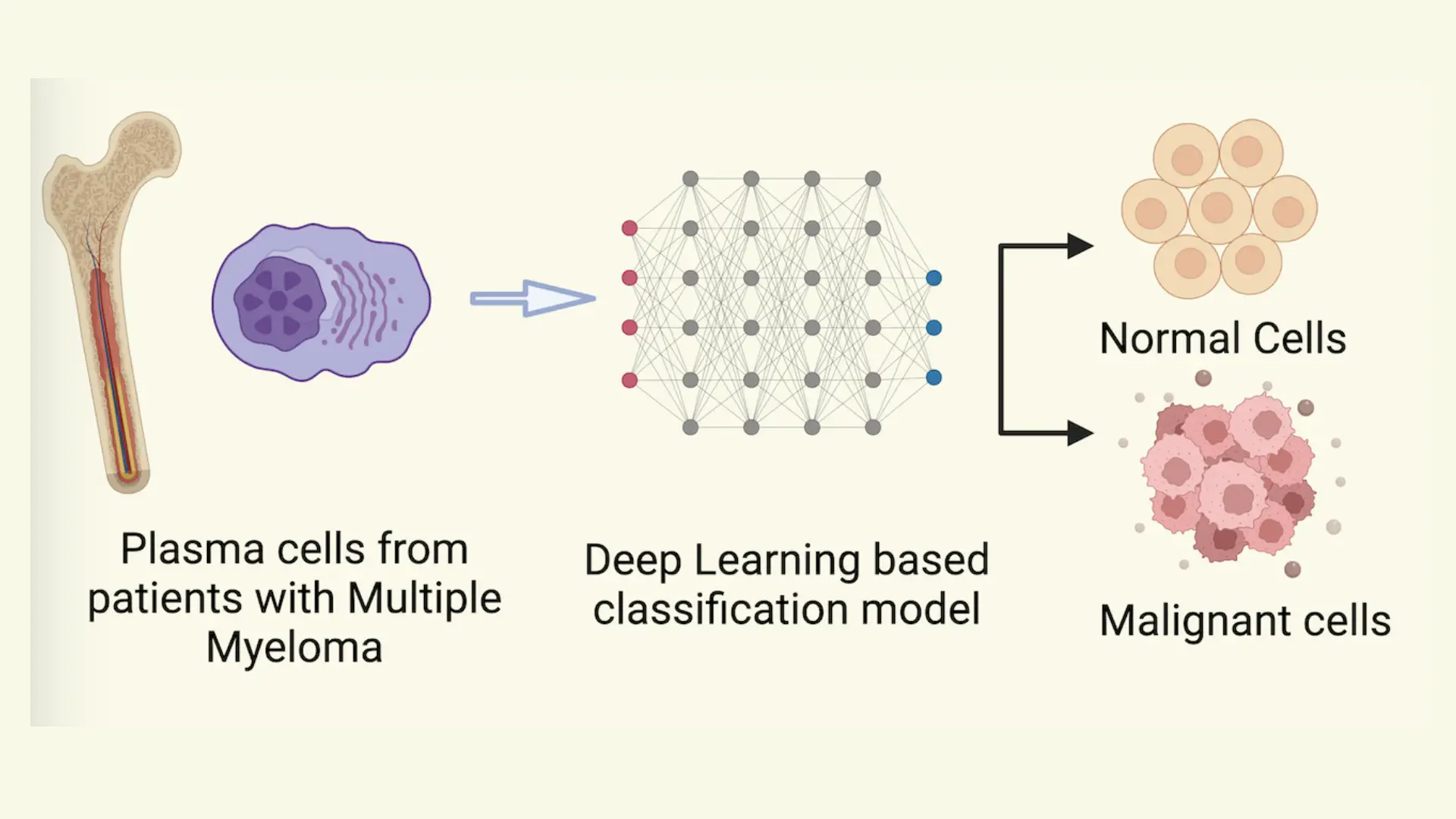
Relevant Publications
NPJ Genomic Medicine, 2023
Despite advancements in understanding the pathophysiology of Multiple Myeloma (MM), the cause of rapid progressing disease in a subset of patients is still unclear. MM's progression is facilitated by complex interactions with the surrounding bone marrow (BM) cells, forming a microenvironment that supports tumor growth and drug resistance. Understanding the immune microenvironment is key to identifying factors that promote rapid progression of MM. To accomplish this, we performed a multi-center single-cell RNA sequencing (scRNA-seq) study on 102,207 cells from 48 CD138- BM samples collected at the time of disease diagnosis from 18 patients with either rapid progressing (progression-free survival (PFS) < 18 months) or non-progressing (PFS > 4 years) disease. Comparative analysis of data from three centers demonstrated similar transcriptome profiles and cell type distributions, indicating subtle technical variation in scRNA-seq, opening avenues for an expanded multicenter trial. Rapid progressors depicted significantly higher enrichment of GZMK+ and TIGIT+ exhausted CD8+ T-cells (P = 0.022) along with decreased expression of cytolytic markers (PRF1, GZMB, GNLY). We also observed a significantly higher enrichment of M2 tolerogenic macrophages in rapid progressors and activation of pro-proliferative signaling pathways, such as BAFF, CCL, and IL16. On the other hand, non-progressive patients depicted higher enrichment for immature B Cells (i.e., Pre/Pro B cells), with elevated expression for markers of B cell development (IGLL1, SOX4, DNTT). This multi-center study identifies the enrichment of various pro-tumorigenic cell populations and pathways in those with rapid progressing disease and further validates the robustness of scRNA-seq data generated at different study centers.
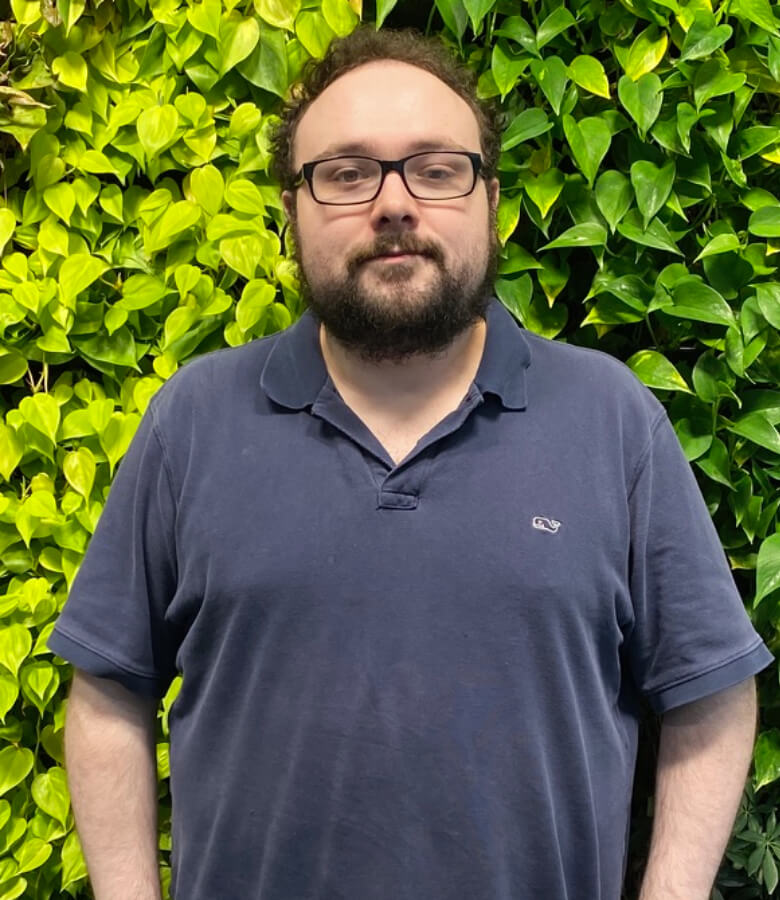
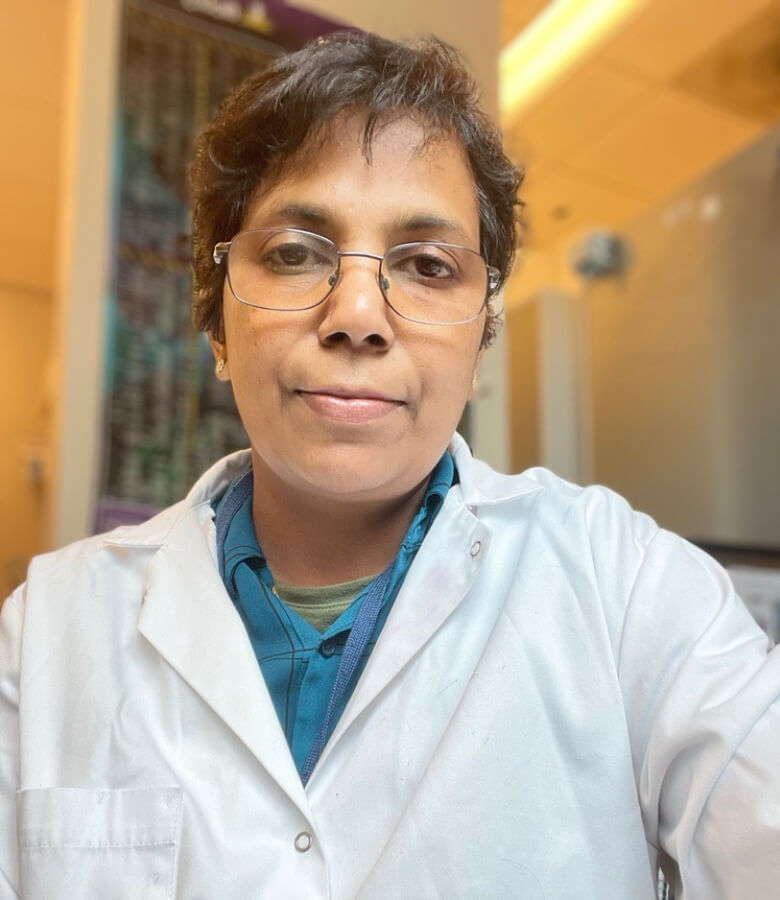
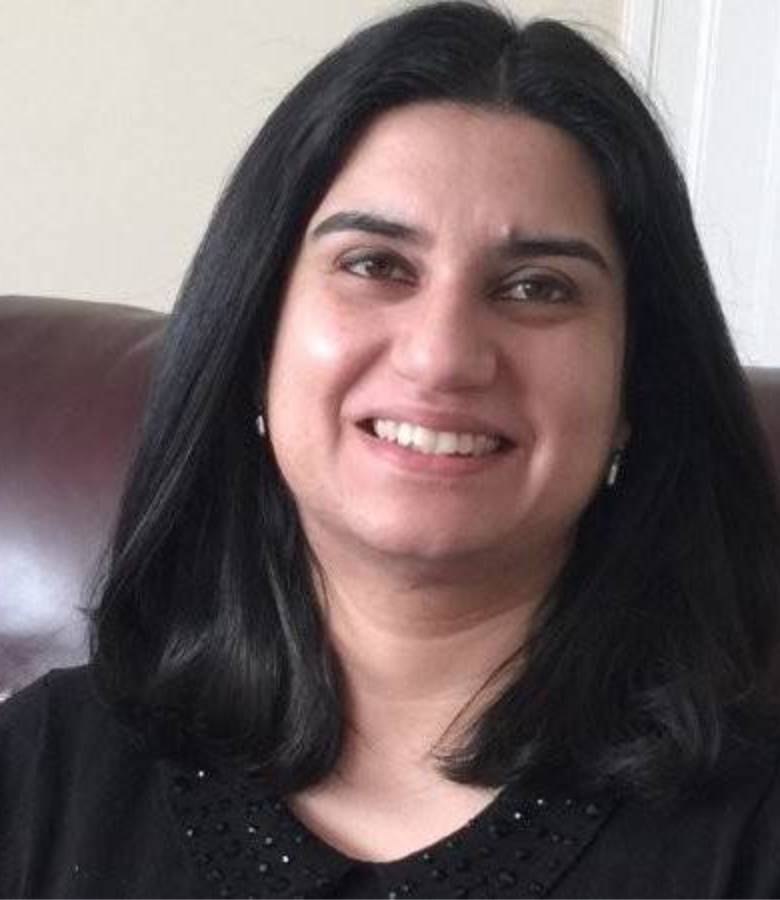
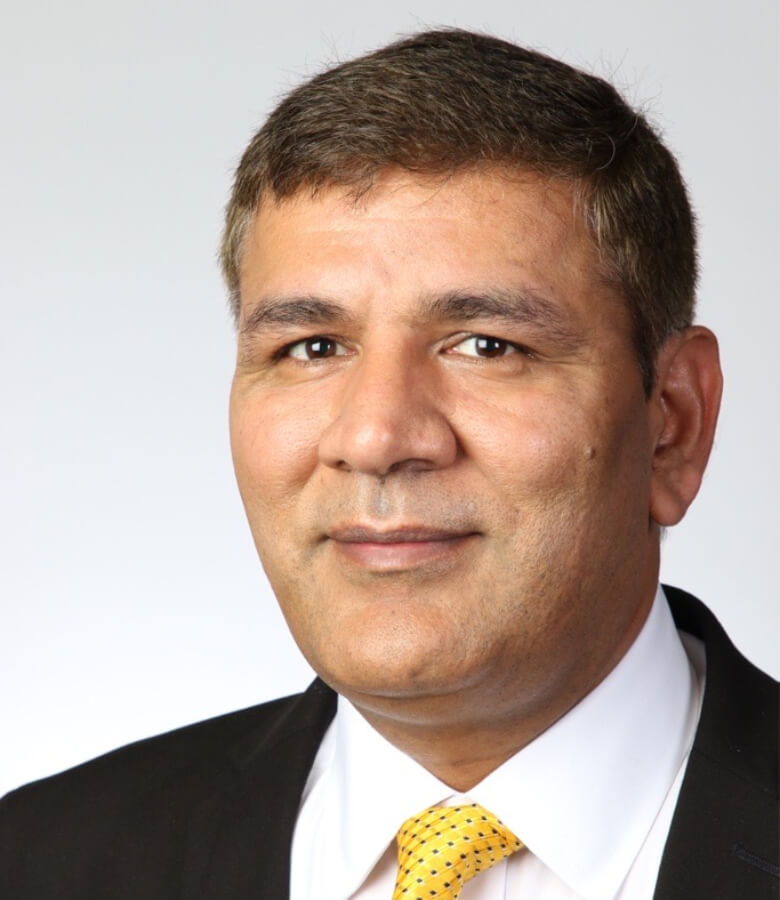
Cancer Research Communications, 2023
As part of the Multiple Myeloma Research Foundation (MMRF) immune atlas pilot project, we compared immune cells of multiple myeloma bone marrow samples from 18 patients assessed by single-cell RNA sequencing (scRNA-seq), mass cytometry (CyTOF), and cellular indexing of transcriptomes and epitopes by sequencing (CITE-seq) to understand the concordance of measurements among single-cell techniques. Cell type abundances are relatively consistent across the three approaches, while variations are observed in T cells, macrophages, and monocytes. Concordance and correlation analysis of cell type marker gene expression across different modalities highlighted the importance of choosing cell type marker genes best suited to particular modalities. By integrating data from these three assays, we found International Staging System stage 3 patients exhibited decreased CD4+ T/CD8+ T cells ratio. Moreover, we observed upregulation of RAC2 and PSMB9, in natural killer cells of fast progressors compared with those of nonprogressors, as revealed by both scRNA-seq and CITE-seq RNA measurement. This detailed examination of the immune microenvironment in multiple myeloma using multiple single-cell technologies revealed markers associated with multiple myeloma rapid progression which will be further characterized by the full-scale immune atlas project.
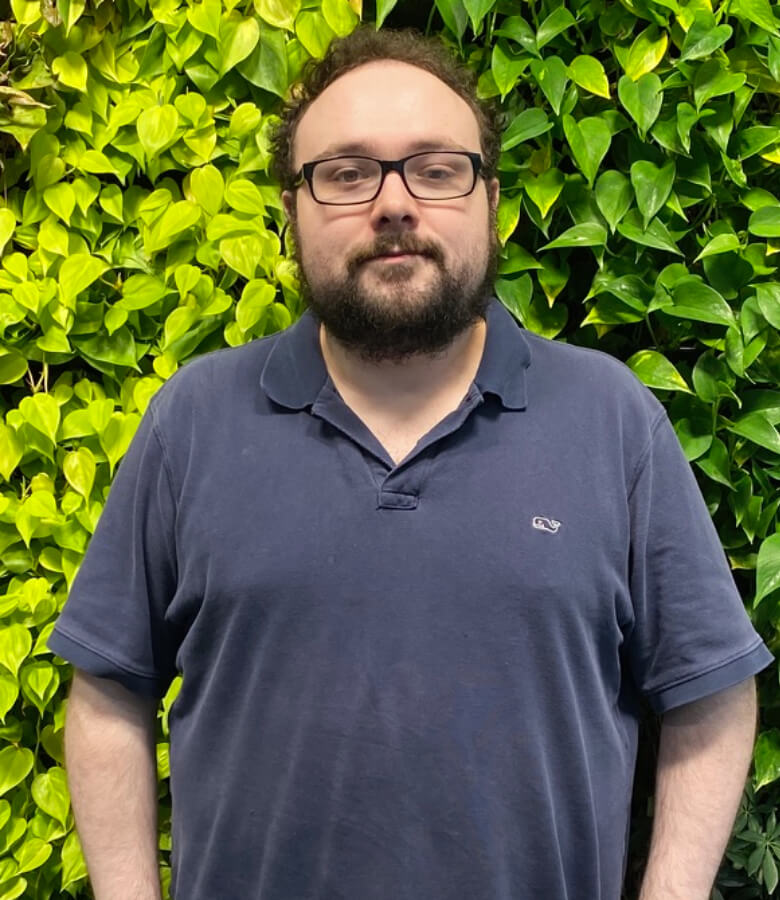
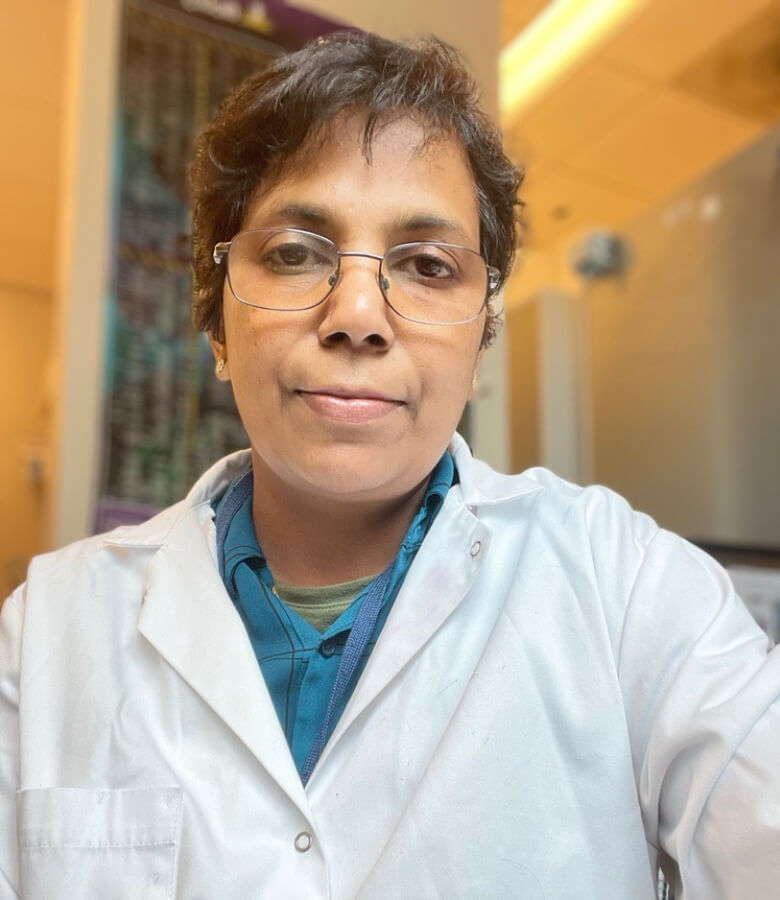
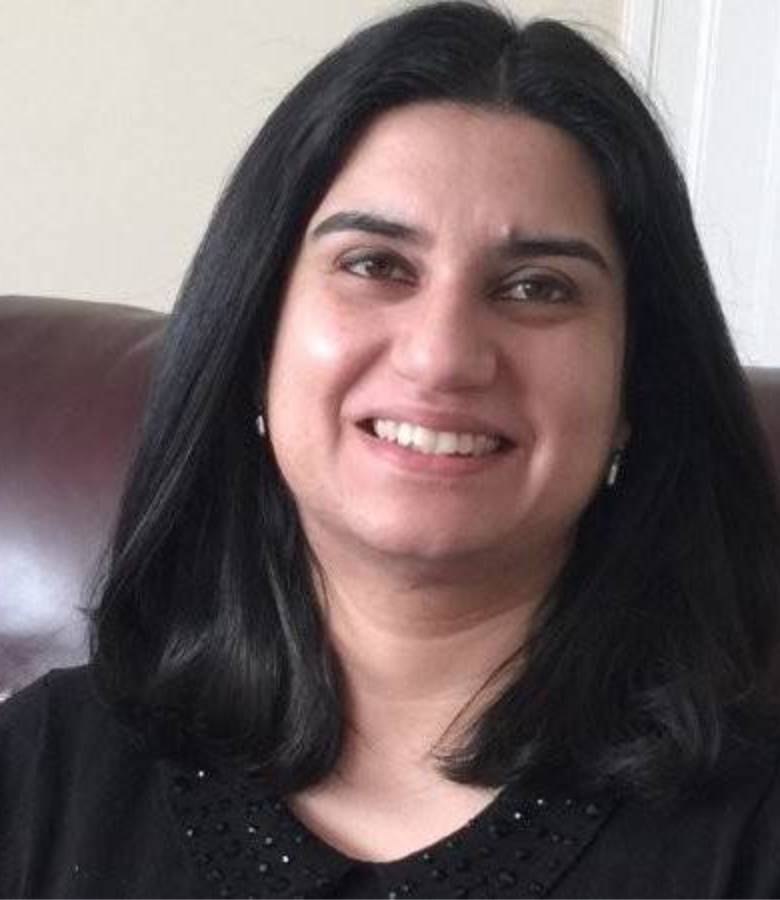
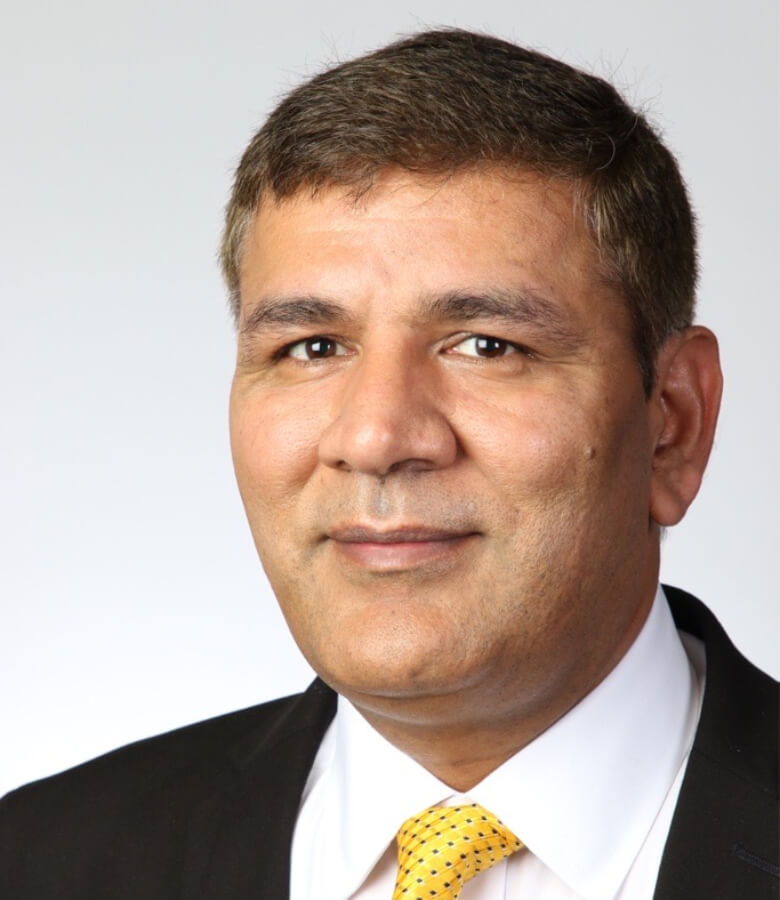
Relevant Presentations
Atlanta Workshop for Single-cell Omics, 2023
Background: Multiple myeloma (MM) is characterized by proliferative plasma cells and our group recently depicted that plasma cells are highly heterogeneous as well as patient-specific by performing single-cell profiling on the bone marrow. Current methods to characterize malignancy in plasma cells rely on marker-based annotation or CNV detection, which require human intervention and are cumbersome. To address this, we implemented a deep learning-based classifier to accurately and automatically identify malignant plasma cells based on single-cell profiles, leveraging its high accuracy and transferable learning ability. Methods: To develop a classifier for differentiating malignant and normal plasma cells, we developed a deep learning- based model. Single-cell RNA sequencing (ScRNA-seq) malignant and non-malignant plasma were obtained from a published study (GSE193531) with 26 subjects, including MM (n=8), smoldering multiple myeloma (SMM) (n=12), and monoclonal gammopathy (MGUS) (n=6), and nine healthy normal bone marrow (NBM) donors. The model architecture includes an autoencoder for dimension reduction and a binary classification unit.
Results: The single-cell RNA-seq data from MM (malignant) and NBM (normal) (n=17) subjects were randomly split into training (60%) and test groups (40%). The deep learning-based model showed high accuracy on both labels on the test data. Training and validating the model on the same patient set increases the variance of the model. Hence, we trained and validated the model on different patient sets of ten and seven subjects, respectively. External validation revealed 97% accuracy of the model in predicting malignant and normal phenotypes. There are two precursor stages in MM: MGUS and SMM. Ground truth labels for these stages were approximated from the gene-expression-based malignancy signature published by the authors of the original dataset. The model was 62% and 83% accurate for MGUS and SMM, respectively.
Conclusions: The deep-learning based model performed well in classifying malignant MM cells from normal plasma cells in NBM. Further testing on the precursor stages depicted slightly lower performance which might be due to the differences in transcriptional states of malignant cells of MM and precursor stages. To further improve the performance of the model in identifying malignant plasma cells from precursor stages of multiple myeloma, we will incorporate more data from plasma cells of MM precursor stages.
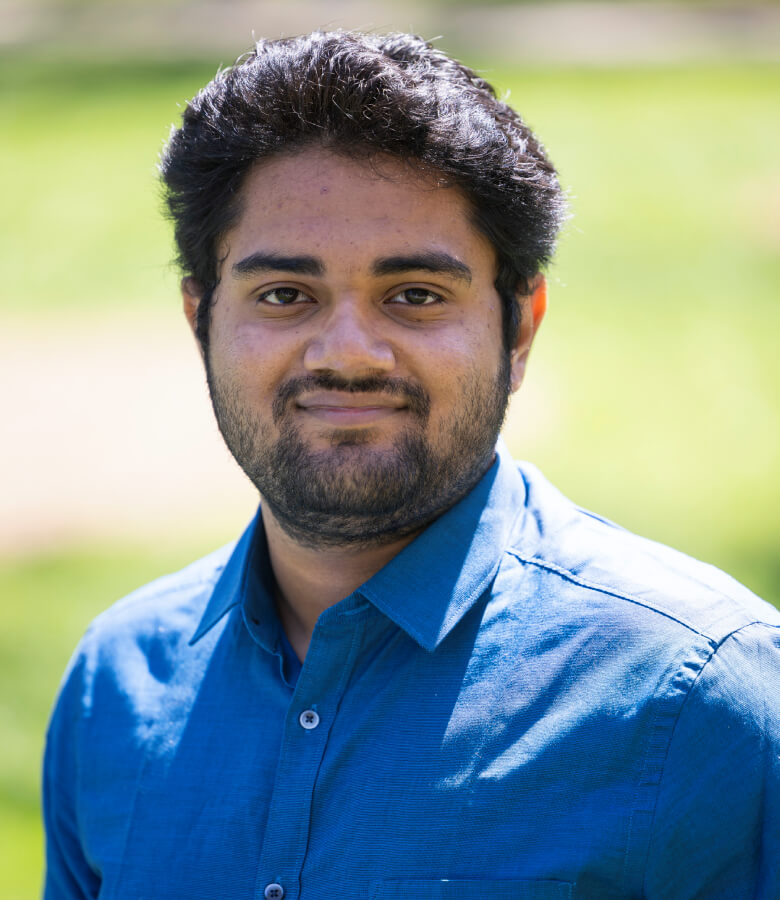
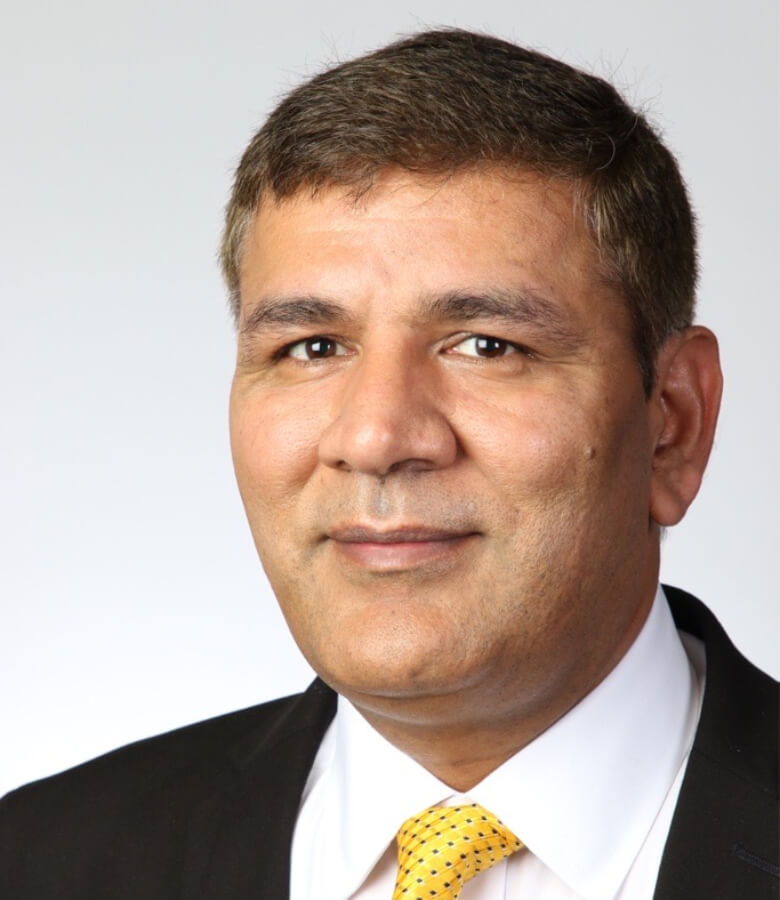
American Society of Hematology Annual Meeting and Exposition, 2021
Introduction: Multiple myeloma (MM) is a complex hematological malignancy with the heterogenous immune bone marrow (BM) environment contributing to tumor growth, drug resistance, and immune escape. T-Cells play a critical role in the clearance of malignant plasma cells from the tumor environment. However, T-Cells in multiple myeloma demonstrate impaired cytotoxicity, proliferation, and cytokine production due to the activation of immune inhibitory receptors from ligands produced by the myeloma cells. In this study, we investigate the behavior of T-Cells in MM patients by using single-cell RNASeq (scRNA-Seq) to compare the transcriptomic proles of BM T-Cells of patients with rapid progressing (FP; PFS < 18mo) and non-progressing (NP; PFS > 4yrs) disease.
Methods: Newly diagnosed MM patients (n=18) from the Multiple Myeloma Research Foundation (MMRF) CoMMpass study (NCT01454297) were identified as either rapid progressors or non-progressors based on their progression free survival since diagnosis. To capture transcriptomic data, scRNA-Seq was performed on 48 aliquots of frozen CD138-negative BM cells at three medical centers/universities (Beth Israel Deaconess Medical Center, Boston, Washington University in St. Louis, and Mount Sinai School of Medicine, NYC). Samples were collected at diagnosis prior to treatment. Surface marker expression for 29 proteins was captured for at least one sample per patient using CITE-Seq. After integration and batch correction, clustering was performed to identify cells of T or NK lineage. Uniform Manifold Approximation and Projection (UMAP) and differential expression were used to identify T-Lymphoid subtypes, and differences in NP and FP samples.
Results: In this study, single cell transcriptomic profiles were identified for ~102,207 cells from 48 samples of 18 MM patients. 40,328 T (CD3+) and NK (CD3-, NKG7+) cells were isolated, and sub-clustered for further analysis (Fig 1A). Using differentially expressed markers for each cluster, the T-Lymphoid subset was split into seven subtypes, consisting of various CD4+ TCells, CD8+ T-Cells, and NK cells (Fig 1B). The CD8+ cells were divided into three distinct phenotypes, namely a GZMK-, GZMB- CD8+ T-Cell cluster, a GZMK+ CD8+ Exhausted T-Cell cluster enriched in TIGIT and multiple chemokines (CCL3, CCL4, XCL2), and a GZMB+ NkT cluster enriched in cytolytic markers (PRF1, GNLY, NKG7) (Fig 1C). Differential expression between NP and FP samples in this CD8+ subset showed enrichment of the NkT cytotoxic markers in NP samples, while FP samples were enriched in the CD8+ Exhausted chemokine markers (Fig 1D). Furthermore, the proportion of CD8+ Exhausted T-Cells was enriched in FP samples (p.val < 0.05) (Fig 1E). Exhaustion markers were measured through both RNA and surface marker levels. In RNA, TIGIT was uniquely associated with the FP-enriched CD8+ Exhausted T-Cell cluster, and CD160 was uniquely expressed in FP samples (Fig 1F). CITE-Seq surface marker expression confirmed enrichment of both TIGIT and PD1 in the CD8+ Exhausted T-Cell cluster, and along with more exhaustion in FP samples (p.val < 0.01).
Conclusions: In this study, we have identified significant differences in T-Cell activity in patients with non-progressing and rapid-progressing multiple myeloma. T-Cells in rapid progressing patients appear to be in a suppressed state, with low cytolytic activity and enriched exhaustion markers. This GZMK+ T-Cell population shows strong similarities with an aging-associated subtype of effector memory T-Cells found to be enriched in older populations (Mogilenko et al, Immunity 54, 2021). These findings will be further validated in an expanded study, consisting both of a larger number of samples, and multiple samples at different timepoints from the same patient.
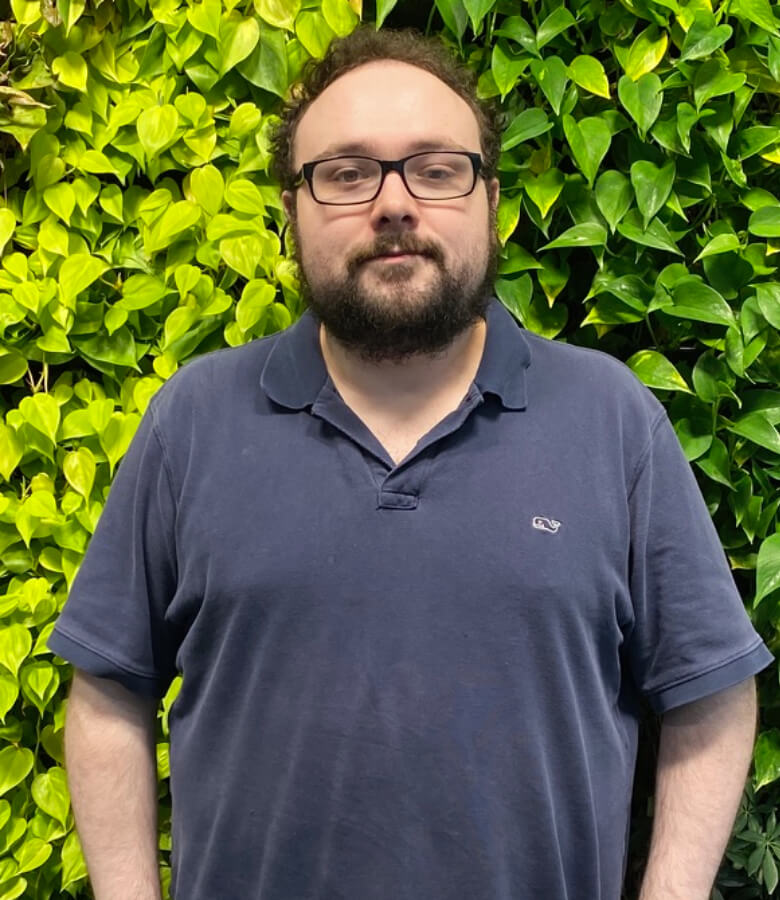
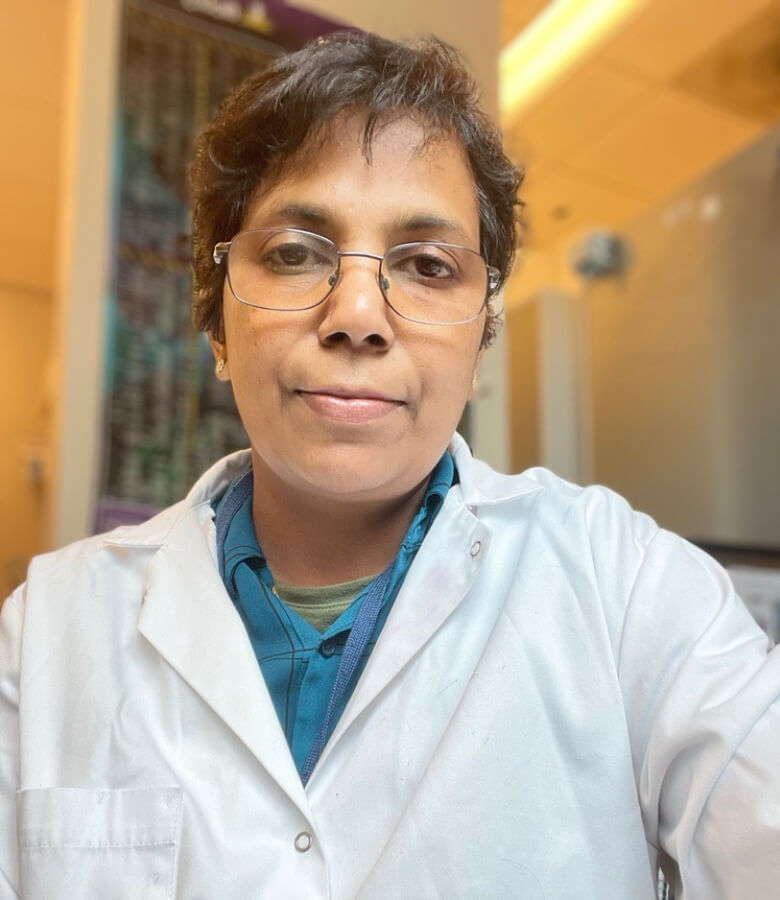
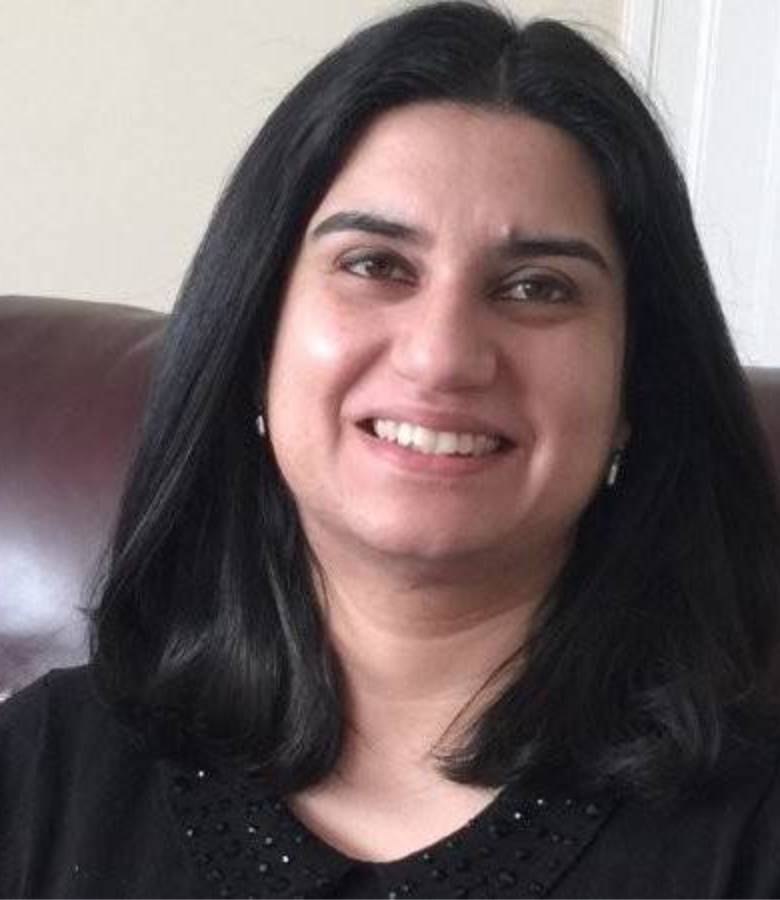
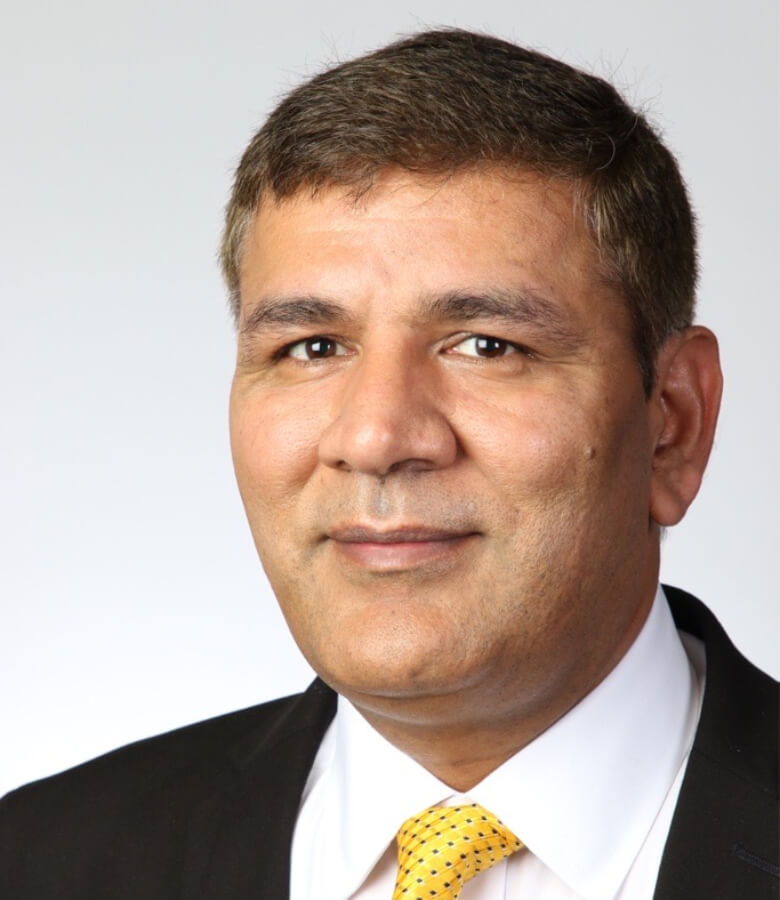